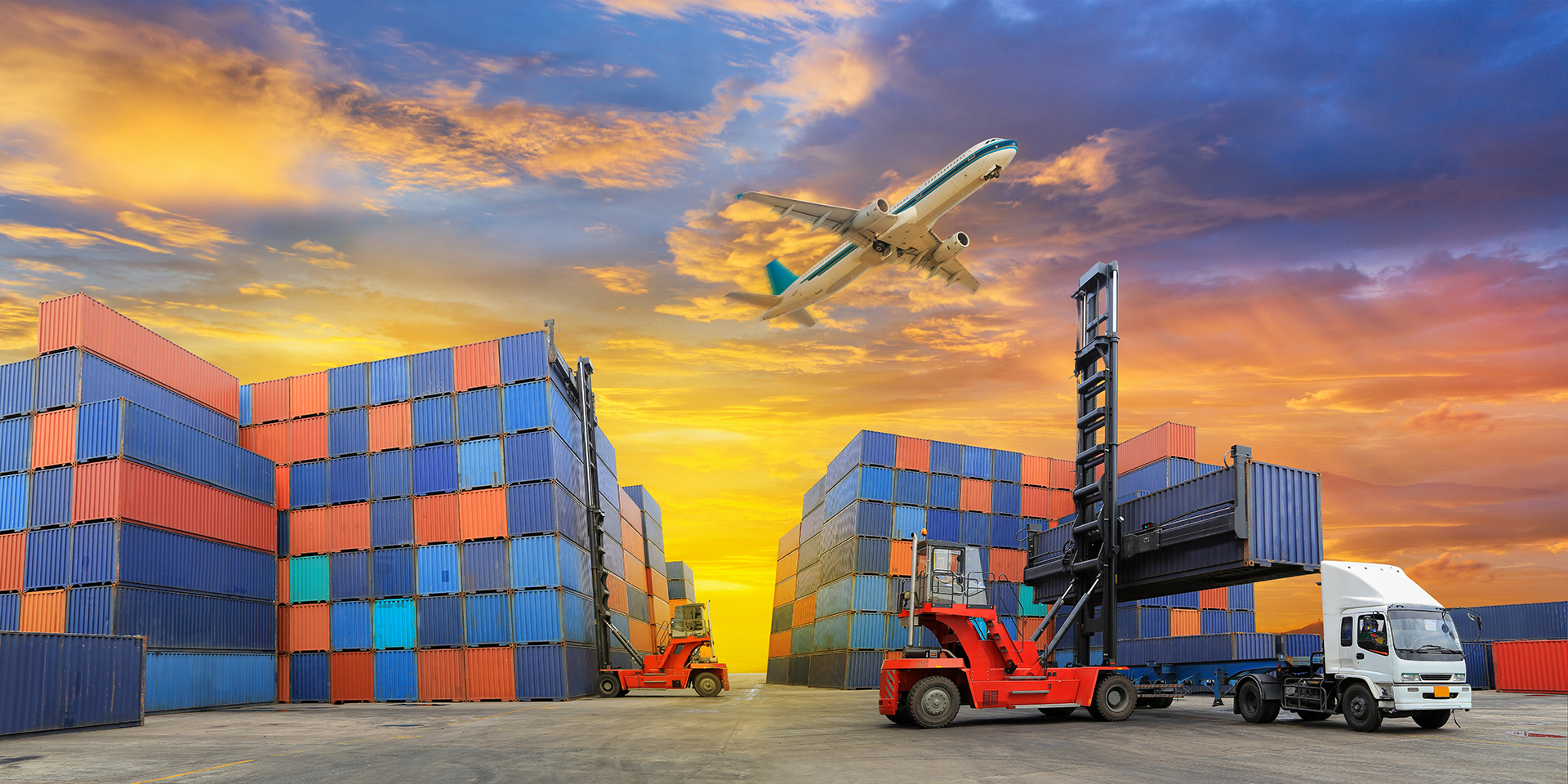
On the Distribution of ML Workloads to the Network Edge and Beyond
The First IEEE INFOCOM Workshop on Distributed Machine Learning and Fog Networks (FOGML 21)
The emerging paradigm of edge computing has revolutionized network applications, delivering computational power closer to the end-user. Consequently, Machine Learning (ML) tasks, typically performed in a data centre (Centralized Learning – CL), can now be offloaded to the edge (Edge Learning – EL) or mobile devices (Federated Learning – FL). While the inherent flexibility of such distributed schemes has drawn considerable attention, a thorough investigation on their resource consumption footprint is still missing. In our work, we consider a FL scheme and two EL variants, representing varying proximity to the end users (data sources) and corresponding levels of workload distribution across the network; namely Access Edge Learning (AEL), where edge nodes are essentially co-located with the base stations and Regional Edge Learning (REL), where they lie towards the network core.
The document is available on IEEE Xplore with the DOI: 10.1109/INFOCOMWKSHPS51825.2021.9484503
https://ieeexplore.ieee.org/document/9484503